Reinforcement Learning: How AI is Learning to Play (and Win) the Game
7 min read
10 Jul 2024
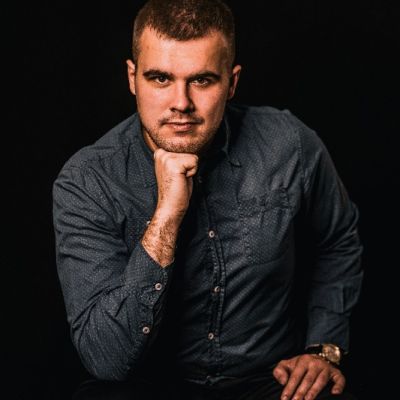
Reinforcement learning (RL) stands at the forefront of AI technologies, enabling machines to learn and make decisions through trial and error interactions with environments. This article explores the principles of reinforcement learning, its applications across various domains, and its potential to revolutionize autonomous decision-making and strategic planning.
Understanding Reinforcement Learning
Reinforcement learning involves an agent learning to navigate an environment to maximize cumulative rewards. Through iterative interactions, the agent learns optimal strategies by receiving feedback (rewards or penalties) based on its actions, enabling it to improve performance over time.

Applications of Reinforcement Learning
Game Playing: Mastering complex games like chess, Go, and video games by learning optimal strategies and decision-making through experience and exploration.
Robotics: Controlling autonomous robots to perform tasks such as navigation, manipulation, and assembly in dynamic and uncertain environments.
Finance: Optimizing portfolio management, algorithmic trading, and risk assessment strategies by learning from historical data and real-time market conditions.
Healthcare: Personalizing treatment plans, optimizing drug dosages, and managing patient care pathways by adapting to individual patient responses and medical conditions.
Benefits of Reinforcement Learning
Adaptive Learning: Continuously improving performance by dynamically adjusting strategies based on real-time feedback and environmental changes.
Complex Decision-Making: Handling intricate decision spaces and uncertain environments where traditional rule-based systems may fall short, offering scalable solutions to complex problems.
Exploration and Exploitation: Balancing exploration of new actions and exploitation of known successful strategies to maximize long-term rewards and achieve optimal outcomes.
Real-World Applications: Applying learned policies and strategies to real-world scenarios, driving autonomous decision-making and operational efficiencies across industries.
Challenges and Considerations
Reward Design: Designing effective reward functions that incentivize desired behaviors and outcomes while avoiding unintended consequences or exploitation of loopholes.
Exploration vs. Exploitation: Striking a balance between exploring new actions and exploiting known strategies to avoid getting stuck in suboptimal solutions or local maxima.
Sample Efficiency: Addressing the need for efficient learning algorithms that minimize data requirements and accelerate convergence in high-dimensional or continuous action spaces.
Future Perspectives
As reinforcement learning advances with innovations in deep neural networks, meta-learning techniques, and policy optimization algorithms, it holds promise for advancing AI capabilities in autonomous systems, decision support tools, and intelligent automation. By enabling machines to learn from experience and optimize behaviors in complex environments, reinforcement learning is driving the evolution of AI towards more adaptive, autonomous, and capable systems.
More Articles

The Quantum Leap: AI & ML Redefining Possibilities
3 min read | 15 Jul 2024

Data Symphony: Harmonizing AI and ML for Innovation
7 min read | 14 Jul 2024

AI Governance: Policies and Regulations for AI Development
4 min read | 13 Jul 2024

AI in Agriculture: Improving Crop Yield and Sustainability
4 min read | 12 Jul 2024
More Articles

Virtual Reality Fitness: Workouts in the Metaverse
6 min read | 28 Aug 2024

Reality Remix: How AR and VR are Blurring the Lines Between Digital and Physical Worlds
3 min read | 27 Aug 2024

Augmented Reality Fashion: Try Before You Buy and Beyond
4 min read | 26 Aug 2024
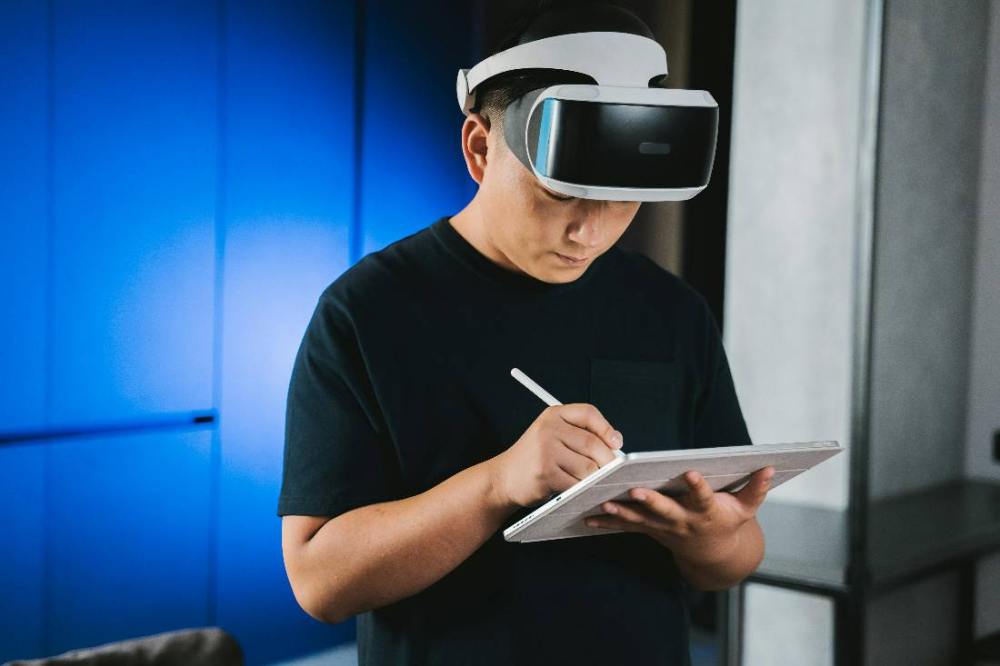
The Art of Storytelling: Immersive Narratives in Augmented Reality
5 min read | 25 Aug 2024