Exploring Explainable AI: Making Machine Learning Models Transparent
5 min read
10 Sep 2024
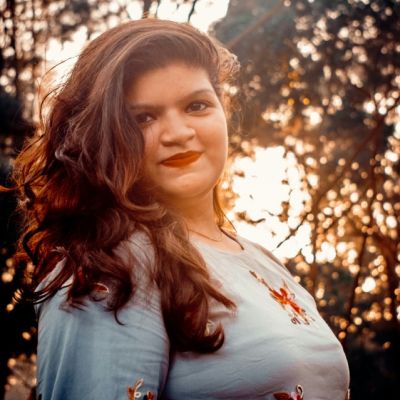
Explainable AI (XAI) is an emerging field focused on making machine learning models more transparent and understandable. As AI systems become more complex, there is a growing need for explanations that clarify how models make decisions and predictions.
One of the primary goals of XAI is to address the "black box" problem associated with many machine learning models. Traditional AI models, especially deep learning algorithms, can be highly opaque, making it challenging to understand how they arrive at specific conclusions. XAI aims to provide insights into the inner workings of these models, enhancing their interpretability and trustworthiness.
Techniques for explainable AI include model-agnostic methods and intrinsic methods. Model-agnostic methods, such as LIME (Local Interpretable Model-agnostic Explanations) and SHAP (SHapley Additive exPlanations), provide explanations for predictions made by any model type. These methods generate local explanations by approximating complex models with simpler, interpretable ones. Intrinsic methods, on the other hand, involve designing inherently interpretable models, such as decision trees or linear regression, which offer more straightforward explanations of their predictions.

Explainable AI is crucial in various domains, including healthcare, finance, and legal systems. In healthcare, for example, transparent AI models can help clinicians understand how diagnostic decisions are made, leading to better patient trust and improved decision-making. In finance, XAI can provide explanations for credit scoring decisions, ensuring fairness and accountability in lending practices.
Despite the benefits, implementing XAI presents challenges. Striking a balance between model complexity and interpretability can be difficult, as more complex models often offer higher performance but less transparency. Additionally, the effectiveness of XAI techniques can vary depending on the specific use case and the nature of the model being explained.
In conclusion, explainable AI is essential for improving transparency and trust in machine learning models. By providing clear and understandable explanations for AI decisions, XAI helps build confidence in these technologies and ensures that they are used responsibly and ethically.
More Articles

The Ultimate Guide to Combining AR and AI for Unbelievable Tech Experiences
6 min read | 06 Sep 2024

Blockchain 2.0: The Breakthrough Innovations That Will Blow Your Mind
7 min read | 05 Sep 2024
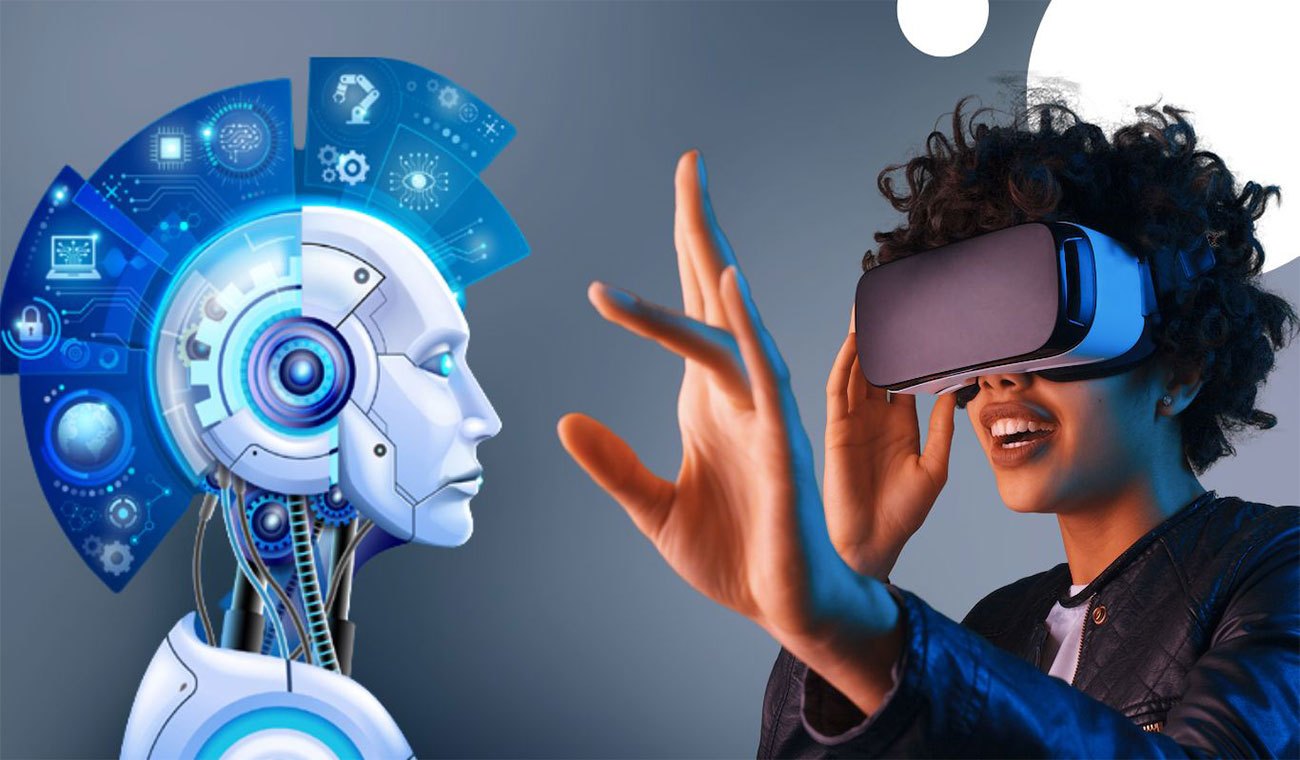
AI Meets VR: How Virtual Reality Is Transforming Machine Learning Training
6 min read | 04 Sep 2024

The AR Revolution: How Augmented Reality Is Changing the Way We Invest in Tech
7 min read | 03 Sep 2024
More Articles

Carbon Capture Technology: Combatting Climate Change
6 min read | 01 Sep 2024

Emotional AI: Machines that Understand Human Emotions
4 min read | 31 Aug 2024

Human Augmentation: Enhancing Abilities with Technology
7 min read | 30 Aug 2024

Photonic Computing: Light-Based Data Processing
7 min read | 29 Aug 2024