Convolutional Neural Networks (CNN): The Secret Behind AI Vision
7 min read
06 Jul 2024
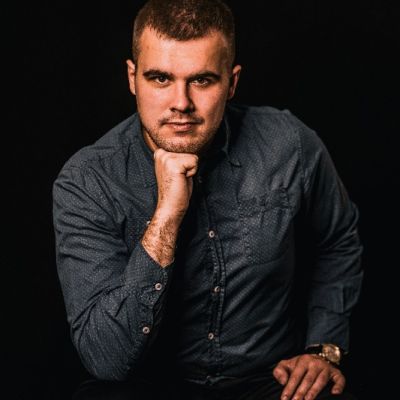
Convolutional Neural Networks (CNNs) have revolutionized the field of computer vision by enabling machines to understand visual data. This article explores the workings of CNNs, their applications across various industries, and their impact on advancing AI-driven visual recognition technologies.
Understanding Convolutional Neural Networks
CNNs are specialized deep learning models designed to process and analyze visual data, such as images and videos. They employ convolutional layers that extract features from input images through filters, pooling layers that downsample feature maps, and fully connected layers that perform classification based on learned features.

Applications of Convolutional Neural Networks
Healthcare: Assisting in medical imaging analysis, disease diagnosis, and surgical planning through accurate image recognition and segmentation.
Automotive: Powering autonomous driving technologies with object detection, lane detection, and pedestrian recognition systems.
Security and Surveillance: Enhancing video surveillance systems with facial recognition, anomaly detection, and activity monitoring capabilities.
E-commerce: Improving product recognition, visual search capabilities, and virtual try-on experiences to enhance customer engagement and sales.
Benefits of Convolutional Neural Networks
High Accuracy: Achieving state-of-the-art performance in image classification, object detection, and image segmentation tasks.
Feature Learning: Automatically learning hierarchical representations of visual features from raw pixel data, eliminating the need for handcrafted feature extraction.
Scalability: Scaling to handle large datasets and complex visual tasks while maintaining computational efficiency and real-time processing capabilities.
Transfer Learning: Facilitating knowledge transfer from pre-trained models to new tasks with minimal additional training data, speeding up model development and deployment.
Challenges and Considerations
Data Quality: Ensuring high-quality and diverse training datasets to prevent biases and improve generalization capabilities of CNN models.
Computational Resources: Managing the computational demands of training and deploying CNNs, especially for real-time applications and large-scale deployments.
Interpretability: Addressing challenges in understanding and interpreting decisions made by complex CNN architectures, especially in critical applications such as healthcare and autonomous driving.
Future Trends
As CNNs continue to evolve with advancements in deep learning techniques, hardware acceleration, and algorithmic improvements, they will play a pivotal role in advancing AI vision capabilities. From enhancing medical diagnostics to enabling smarter cities, CNNs are poised to drive innovation and transform industries by unlocking new possibilities in visual data analysis and interpretation.
More Articles

AI and Space Exploration: Enhancing Missions with Intelligent Systems
7 min read | 14 Aug 2024

Bias in AI: Addressing Fairness and Inclusivity in Machine Learning Models
5 min read | 13 Aug 2024

The Role of AI in Drug Discovery and Development
6 min read | 12 Aug 2024
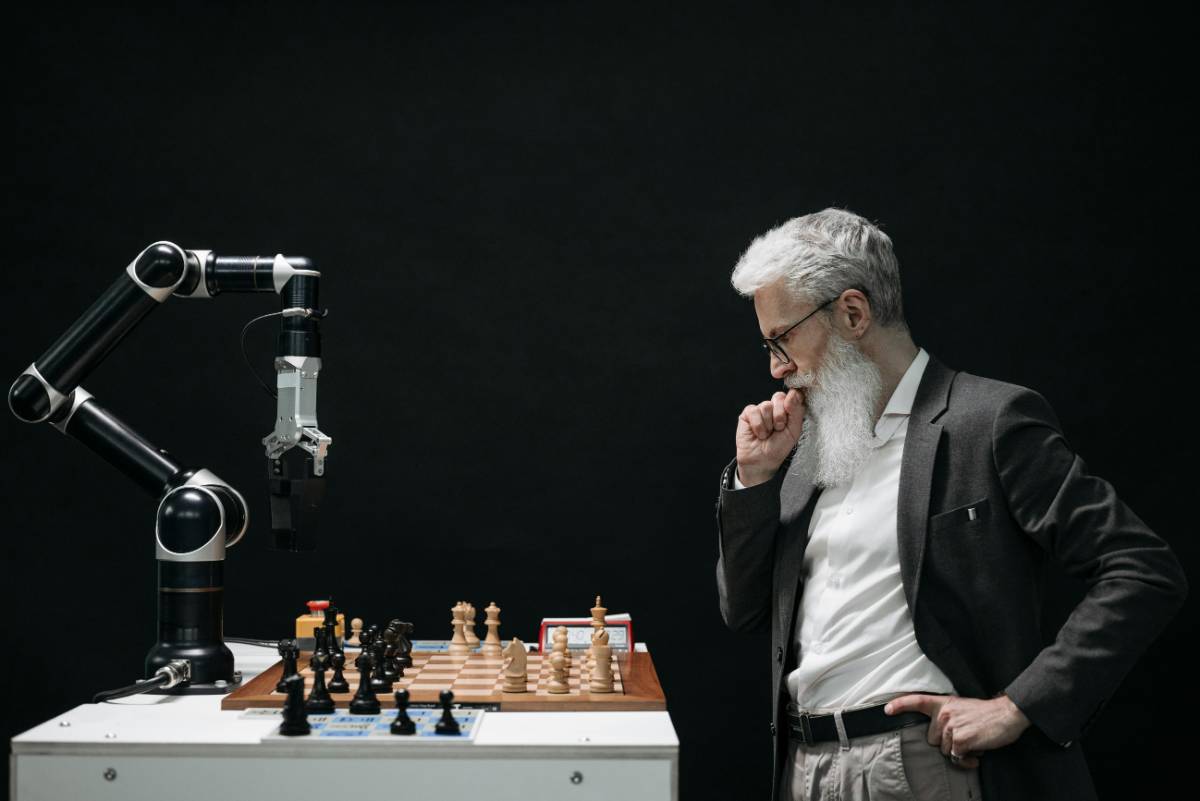
AI in Sports: Performance Analysis and Injury Prevention
7 min read | 11 Aug 2024
More Articles

The Intersection of AR and AI: Creating Smarter Augmented Experiences
5 min read | 04 Sep 2024
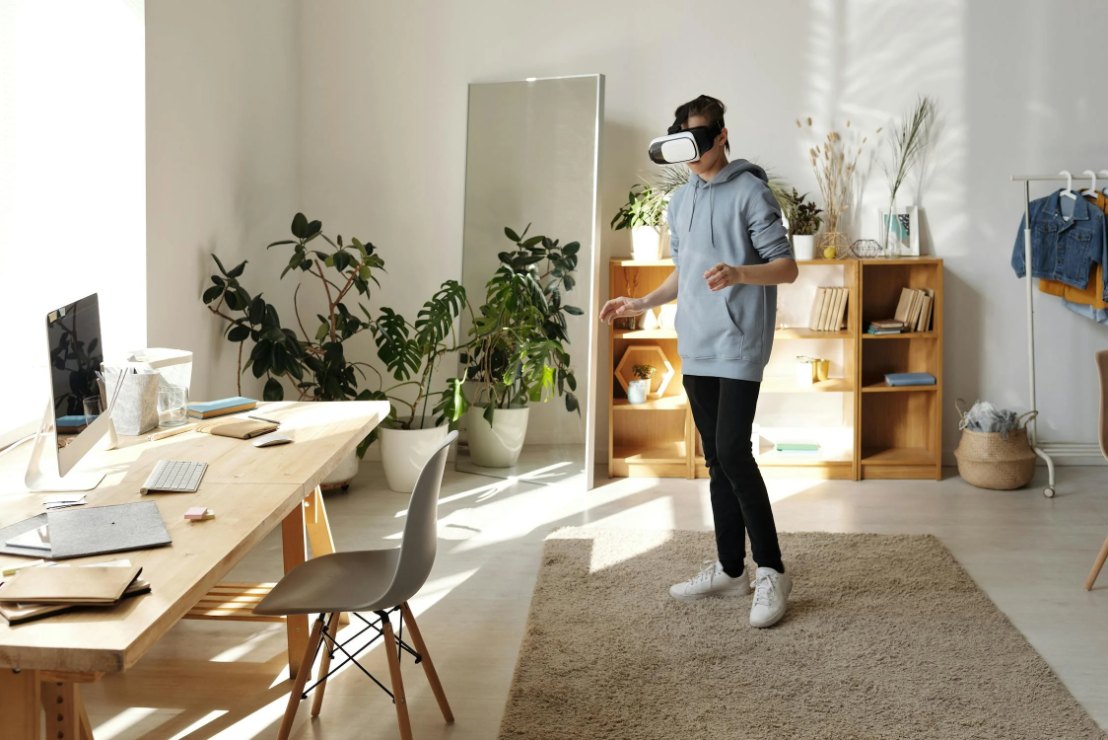
Using VR for Remote Collaboration: How Virtual Reality Is Bridging Distance Gaps
6 min read | 03 Sep 2024

The Evolution of AR Glasses: What to Expect from the Next Generation
6 min read | 11 Sep 2024

How VR is Shaping the Future of Travel: Virtual Destinations and Experiences
5 min read | 10 Sep 2024